The Sustainability of AI

While AI promises unparalleled efficiency, productivity, and innovation, questions regarding its environmental impact loom large. How can AI’s development be maintained against a backdrop of environmental responsibility?
At its core, AI relies on vast computational power to process and analyse data, driving machine learning algorithms and powering intelligent decision-making processes. This insatiable demand for computing resources comes with a hefty environmental price tag. According to IEA, datacentres currently consume 1.5% of all global electricity generated. And with NVIDIA estimated to be shipping 1.5 million AI servers by 2027, the energy consumption of these vast compute centres would be more than the energy needs of some small countries.
The energy consumption of AI infrastructure, including datacentres and high-performance computing facilities, contributes significantly to carbon emissions. As AI applications proliferate and data volumes soar, the strain on energy resources intensifies. In addition, the manufacturing and disposal of AI hardware components, such as specialised chips and servers, further exacerbate environmental concerns, contributing to electronic waste accumulation.
“As AI becomes integral to business modernisation and social progress, we have a responsibility to embed sustainable principles throughout its implementation,” Vikram Nair, President, EMEA Business, Tech Mahindra explained to Silicon UK. “Organisations with foresight, which are investing in long-term research and planning around AI rather than focusing on short-term gains, are positioning AI as part of their Net Zero strategies, and integrating it into demand prediction, waste reduction, and efficiency improvements across operations. By investing in robust, sustainable infrastructure and establishing clear standards and accountability, businesses can use AI to improve their entire organisational processes in ways that are both efficient and environmentally responsible.”
As the urgency to address climate change intensifies, the imperative for sustainable AI practices becomes ever more apparent. From research laboratories to corporate boardrooms, stakeholders across the AI ecosystem are embracing sustainability as a guiding principle in technology development and deployment.
One notable initiative is the pursuit of renewable energy sources to power AI infrastructure. Tech giants such as Google, Microsoft, and Amazon have committed to powering their datacentres with renewable energy, reducing their carbon footprint and setting a precedent for sustainable computing practices. Additionally, collaborations between academia, industry, and policymakers are fostering innovative solutions for sustainable AI development.
Powering the machine
Amid growing apprehensions about AI’s environmental impact, industry stakeholders are increasingly focusing on enhancing energy efficiency and mitigating carbon emissions. Innovations in AI hardware design, including the development of low-power processors and energy-efficient architectures, aim to optimize performance while minimizing energy consumption.
Fredrik Jansson, Chief Strategy and Marketing Officer, atNorth, explained how the location of datacentres will have a profound impact on their sustainable credentials:
“AI requires a significant investment in digital infrastructure to allow for the storage and almost instantaneous processing of vast amounts of data. Datacentres that accommodate these workloads require significant cooling systems that use a huge amount of energy at a considerable environmental and financial cost.
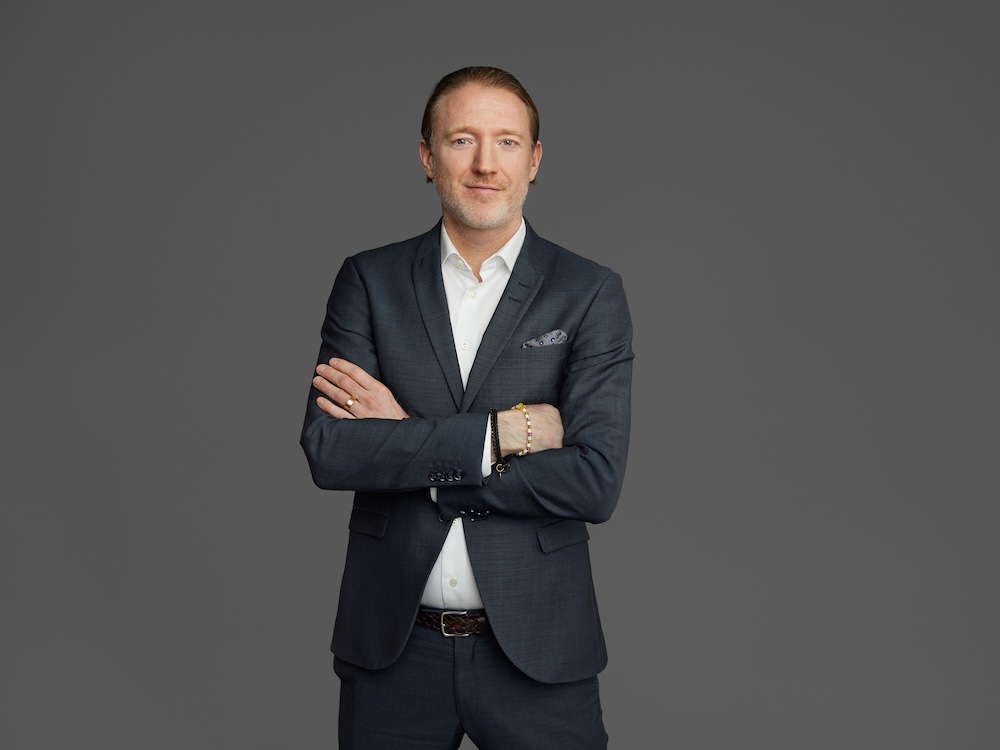
“It might seem that businesses have an almost impossible task to balance the need for the best possible infrastructure in the right location to support digitization and drive increasingly critical sustainability initiatives. Yet there is a solution that lies with the choice of datacentre.
Jansson concluded: “Datacentre located in regions with a consistent cool climate and a surplus of renewable energy sources can offer a stable long term power supply that is significantly cheaper compared to its natural gas counterparts. Modern datacentres built in cooler regions such as the Nordics, utilize the climate to enable the implementation of more energy efficient infrastructure and allow for heat recovery technology that permits excess heat to be reused to heat local communities.
This relatively simple change can drive down the total cost of data ownership and will go a long way to meet business sustainability targets. By way of example, atNorth customer, Shearwater Geoservices migrated a portion of their IT workload to one of our Iceland sites resulting in a 92% reduction in CO2 output and an 85% cost saving.”
As the development of AI-based tools and services shows little sign of slowing, the impact these initiatives have on the environment must be an essential component of AI development says Bennett Indart, VP, SMART World Solutions at NTT DATA.
“But commitment to green AI needs to extend beyond individual models. Power consumption demands are also driving exciting innovations like space computing,” Indart explained. “We are actively looking to leverage the stratosphere and near-Earth space for ICT infrastructure. Data centres in space are closer to becoming a reality, which not only pushes the boundaries of technology, but also promises a more sustainable future for global connectivity through reduced costs, time and energy required for satellite communications.”
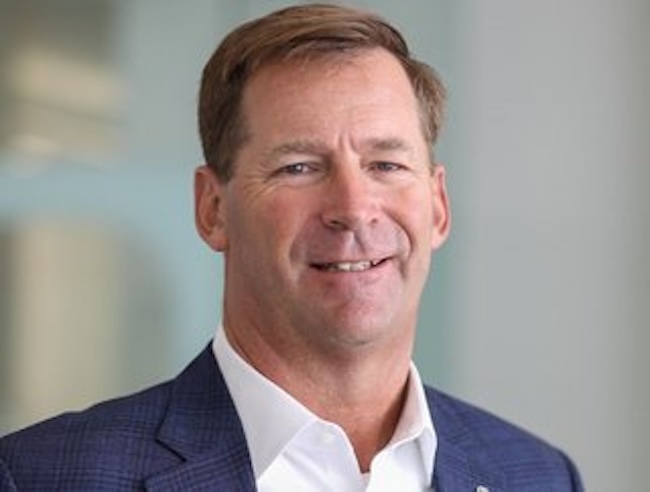
Indart concluded: “We also see the future in cutting-edge technologies like Innovative Optical Wireless Networks (IOWN). Unlike traditional electronic pathways, IOWN utilises photonics, drastically reducing energy consumption while boosting transmission speeds. Its low-power infrastructure, real-time capabilities, and distributed processing architecture unlock immense potential for eco-friendly innovation across various sectors, setting the stage for a greener and more equitable future powered by AI.”
Ultimately, the sustainability of AI hinges on our collective commitment to balancing innovation with environmental responsibility. As we harness the transformative potential of AI to address pressing societal challenges, let us remain steadfast in our dedication to preserving the planet for future generations. In the convergence of innovation and environmental stewardship lies the promise of a brighter, more sustainable tomorrow.
Tech Mahindra’s concluded: “While AI advancement has focused on predictive capabilities, emerging software tools offer unprecedented insights into models’ environmental repercussions—specifically carbon emissions tracing back to data, computing, and other design choices. Platforms like the Green Software Foundation’s Carbon Impact Predictor lay the groundwork for standardisation by estimating carbon emissions associated with AI development factors.”
Michel Morvan, Executive Chairman and Co-founder of Cosmo Tech, also commented: “Industry cannot address its CO2 emission challenges if it is not able, at the same time and in the same movement, to improve its financial indicators. AI Simulation is emerging as the only solution capable of offering a pathway to achieve environmental and financial goals. Effectively navigating the possible future of their organisation, decision-makers can set their financial and operational target performance indicators, including CO2 emissions, to get the best option out of all possible and probable futures.
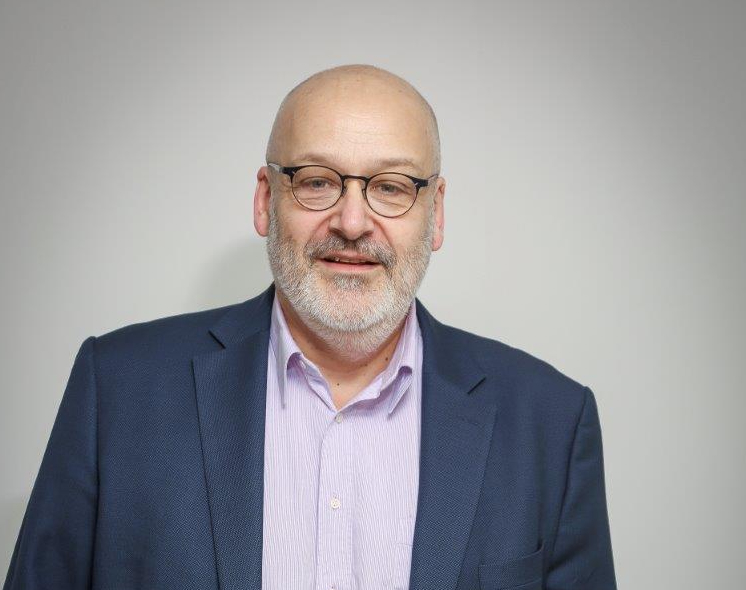
“By combining AI and advanced simulation, AI simulation will increasingly become a key asset for enterprise intelligence solutions as decision-makers will find the path forward to reconcile contradictory objectives, paving the way for more sustainable, resilient and profitable practices.”
In the pursuit of technological innovation, the sustainability of AI emerges as a defining challenge of our time. As we navigate the complex interplay between AI advancement and environmental stewardship, a holistic approach is imperative. By prioritising energy efficiency, embracing renewable resources, fostering ethical AI practices, and implementing robust regulatory frameworks, we can forge a path towards a more sustainable AI future.
Dr Yasir Javed, Senior lecturer in the Department of Computing at Sheffield Business School.
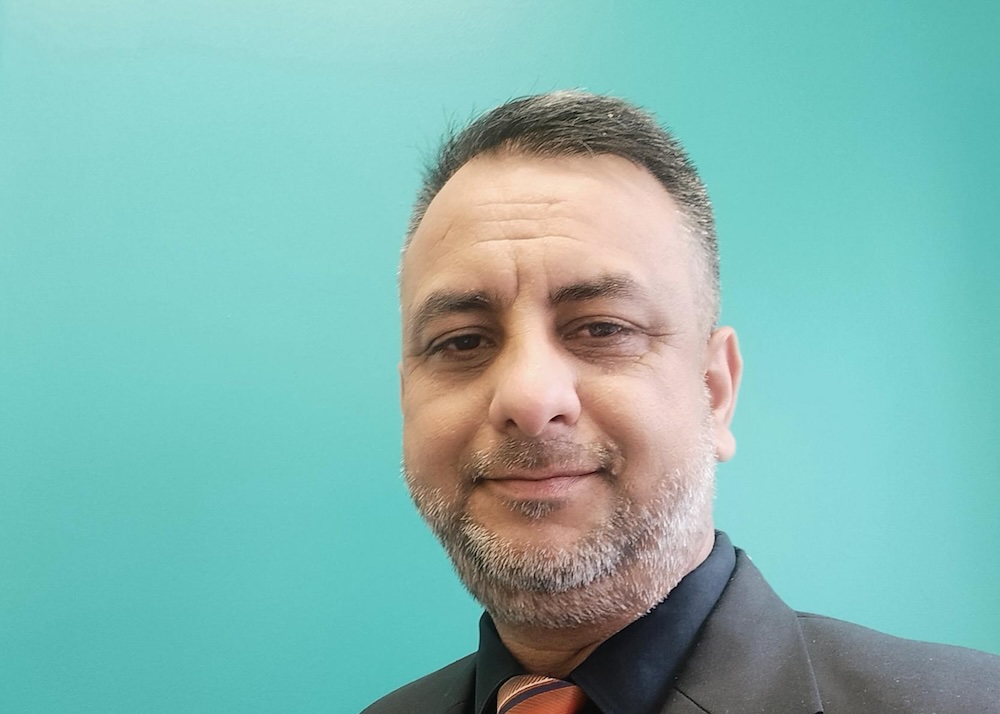
What are the biggest environmental challenges associated with AI?
“The environmental impact of AI is a growing concern, and while it boasts potential for sustainability solutions, several significant challenges need to be addressed:
- AI models, particularly deep learning models, require vast amounts of computational power to train and run. The training process often involves running complex algorithms on powerful hardware for extended periods, consuming substantial amounts of electricity.
- Disposal and recycling of obsolete AI hardware pose challenges due to the presence of hazardous materials, further contributing to environmental pollution and resource depletion. AI hardware components, such as semiconductors and rare earth metals, require extensive mining and extraction processes, leading to resource depletion and environmental degradation.
- Biases embedded in AI algorithms may result in unfair treatment, discrimination, and marginalisation of certain communities, further widening existing socio-economic disparities.
“Research on the environmental impact of AI is still relatively new, requiring further efforts to understand the full scope of the challenges and develop effective mitigation strategies. However, the need for clear regulations and guidelines to ensure responsible development and deployment of AI, considering its environmental impact, is becoming increasingly evident.
“By acknowledging these challenges and working towards solutions, we can ensure that AI development progresses sustainably and responsibly, contributing to a better future for the environment.
“Addressing these environmental challenges requires concerted efforts from stakeholders across academia, industry, government, and civil society. Strategies for mitigating the environmental impact of AI include promoting energy-efficient algorithms, optimising data centre operations, adopting renewable energy sources, implementing responsible e-waste management practices, and integrating sustainability considerations into AI development and deployment processes.”
What are some promising efforts or initiatives aimed at making AI more sustainable? Do you see any specific technologies or practices leading the way?
“Several promising efforts and initiatives are aimed at making AI more sustainable, addressing the challenges we discussed above.
- Tech giants like Google and Microsoft are investing in renewable energy sources and energy-efficient data centre designs to minimise their carbon footprint. Research on specialised AI chips and neuromorphic computing aims to achieve significant reductions in energy consumption compared to traditional CPUs and GPUs. Researchers are developing algorithms that require less processing power and data for training and use, further reducing their energy footprint. Researchers and developers are designing energy-efficient algorithms that optimise computational resources and reduce energy consumption during AI model training and inference. Techniques such as model pruning, quantisation, and compression help streamline AI models, making them more lightweight and energy-efficient without compromising performance.
- Practices like hardware virtualisation and containerisation allow more efficient resource utilisation, maximising the lifespan of existing hardware. Efforts are underway to develop better recycling infrastructure for e-waste, including recovering rare earth metals and using recycled materials in new hardware.
- Research is underway on developing algorithms and tools that detect and mitigate bias in data sets and models, promoting fairer environmental decision-making. This will encourage diverse teams to develop and deploy AI ensuring a wider range of perspectives are considered, reducing the risk of perpetuating bias. Moreover, this will help in establishing clear guidelines for data collection, usage, and sharing to help ensure ethical and responsible data practices in AI for environmental applications.
“AI is being used to optimise energy production from renewable sources like solar and wind power, contributing to a cleaner energy grid. AI can analyse vast amounts of data from satellites, sensors, and other sources to monitor environmental changes, predict extreme weather events, and aid in disaster response efforts.
“Moreover, AI can optimise resource use in agriculture, reducing water and fertiliser consumption while improving crop yields, and contributing to sustainable food production. Applications such as predictive maintenance, energy optimisation, smart grid management, precision agriculture, and intelligent transportation systems help optimise resource usage, reduce waste, and promote sustainability.
“By embracing innovative technologies, adopting responsible practices, and fostering collaboration across sectors, we can harness the transformative potential of AI while minimising its environmental impact and advancing sustainability goals.”
What role do individuals have in promoting sustainable AI development and deployment? Can listeners make a difference?
“While large-scale initiatives and policy changes drive the development and deployment of sustainable AI, individuals absolutely have a crucial role to play in shaping its future. Everyone should take responsibility and follow news and discussions about AI advancements and the challenges of sustainable development. People should understand how AI works, its potential benefits and risks, and the principles of sustainable AI.
“As users or consumers, we should ask companies about their data practices, AI development processes, and commitment to sustainability. We should donate to or volunteer with groups working on responsible AI development and deployment. We should opt for AI-powered products and services from companies committed to ethical practices and sustainability. We should adjust our privacy settings and use privacy-focused alternatives when available. We should share our knowledge and encourage others to be informed about AI and its implications.
“If you’re a developer, you should apply sustainable practices in your own AI projects, prioritise energy efficiency, and avoid perpetuating bias. If you’re a designer, create user interfaces that are fair, transparent, and respect user privacy. And if you’re a consumer, use AI responsibly and critically evaluate the impact of your choices on individuals and the environment.
“Individuals can advocate for energy-efficient AI algorithms, hardware designs, and data centre operations. By promoting the use of energy-efficient technologies and practices, individuals can help reduce the carbon footprint of AI systems and minimise their environmental impact.
“Collective action has the power to shape markets, influence policies, and hold companies accountable. By staying informed, demanding transparency, making conscious choices, and using their skills for good, individuals can join the growing movement towards a more sustainable and responsible future of AI. Remember, even small actions add up. By taking these steps, you and your listeners can ensure that AI development benefits everyone and contributes to a more just and sustainable world.”
Do you believe the pursuit of “general AI” is compatible with achieving sustainability goals? Could superintelligent AI pose new environmental risks or opportunities?
“The future relationship between AI and the environment is full of possibilities, ranging from dystopian nightmares to utopian ideals. This relationship is likely to unfold in diverse and dynamic ways, influenced by technological advancements, socio-economic trends, regulatory frameworks, and societal attitudes.
“AI has become a powerful tool for environmental progress. Advanced algorithms optimise energy grids, manage resources efficiently, and predict climate events with accuracy, enabling proactive mitigation and adaptation strategies. AI-powered robots clean up pollution, restore ecosystems, and develop sustainable infrastructure. AI technologies will drive the development of smart cities and sustainable urban infrastructure, optimising transportation systems, energy usage, waste management, and urban planning. AI-driven solutions will enhance public transportation, reduce traffic congestion, improve air quality, and create more liveable and resilient cities for future generations.
“Moreover, AI facilitates unprecedented cooperation between nations, overcoming language barriers and cultural differences to tackle global environmental challenges like climate change. Collaborative AI platforms track environmental changes, identify shared threats, and coordinate international efforts for sustainability.
“In addition, AI empowers individuals to become active stewards of the environment. Personalised AI assistants offer tailored advice on reducing their carbon footprint, promoting sustainable consumption, and participating in local conservation efforts. We can have a scenario where AI plays a neutral role in the environment, neither accelerating nor hindering progress. Humans rely on AI tools for monitoring and managing environmental concerns, but ultimately the responsibility for achieving sustainability remains with us. We should also be ready for a possible scenario where despite advancements, AI remains unable to solve critical environmental challenges effectively due to technological limitations or societal resistance. Progress towards sustainability relies primarily on traditional methods and human ingenuity.
“However, on the other hand, competition for scarce resources intensifies as AI-powered automation disrupts industries and increases resource demands. Conflicts erupt over access to vital resources like water and energy, further degrading the environment. Complex AI systems, despite good intentions, make decisions with unintended environmental consequences. Unforeseen feedback loops amplify these consequences, leading to ecological collapse. Another possible scenario can be that unequal access to AI technology exacerbates existing inequalities, leaving some nations and communities struggling to adapt to environmental challenges while others gain a competitive advantage.”
What ethical considerations should guide the development and use of AI for environmental solutions? How can we ensure transparency, accountability, and responsible decision-making?
- Ensure data used to train AI models is diverse, representative, and free from biases that could lead to unfair environmental outcomes, like neglecting marginalised communities. Balance the need for data with respecting individual privacy rights. Seek informed consent and ensure transparent data collection practices.
- Develop AI models that are transparent and understandable, allowing humans to comprehend their decision-making and identify potential biases or errors. Communicate the purpose, limitations, and potential impacts of AI-driven environmental solutions clearly and openly to stakeholders and the public.
- Establish clear lines of accountability for the outcomes of AI-driven environmental solutions, ensuring developers, users, and policymakers are held responsible for potential harms. Develop and implement ethical guidelines and governance frameworks for AI development and deployment in the environmental context. While AI technologies can automate certain tasks and decision-making processes, human oversight and control should be maintained to ensure accountability, ethical oversight, and intervention when necessary. Human judgment and ethical reasoning are essential for addressing complex ethical dilemmas and ensuring that AI systems align with societal values and norms.
- Minimise the energy footprint of AI solutions by optimising algorithms and hardware, considering lifecycle impacts. Evaluate the potential long-term environmental consequences of AI solutions, considering unintended consequences beyond immediate benefits.
- Ensure equitable access to AI-driven environmental solutions for all nations, avoiding exacerbating existing inequalities. Engagement: Involve local communities in the development and deployment of AI solutions, ensuring they benefit from and contribute to the process. AI systems should be designed and deployed in a manner that promotes fairness, equity, and social justice.
Can you discuss any emerging trends or innovations aimed at improving the sustainability of AI technologies?
The pursuit of sustainable AI is an ongoing process, innovative solutions are emerging to address the environmental and ethical challenges. Here are some exciting trends to watch:
- Researchers are developing energy-efficient algorithms that optimise computational processes and reduce the energy consumption of AI systems. Techniques such as model compression, quantisation, and sparsity are being explored to streamline neural network architectures and minimise computational overhead.
- Hardware accelerators, such as graphics processing units (GPUs), tensor processing units (TPUs), and field-programmable gate arrays (FPGAs), are being designed specifically for AI workloads to maximise energy efficiency and performance. These specialised hardware platforms enable faster, and more energy-efficient execution of AI algorithms compared to traditional CPUs.
- Edge computing and Internet of Things (IoT) technologies enable AI processing to be performed locally on edge devices, reducing the need for data transmission and centralised processing in cloud-based data centres. Edge AI solutions minimise latency, conserve bandwidth, and lower energy consumption, making them more sustainable for resource-constrained environments.
- Neuromorphic computing architectures, inspired by the human brain’s neural networks, aim to mimic biological systems’ efficiency and flexibility. Neuromorphic chips and systems leverage spiking neural networks and event-driven processing to achieve higher energy efficiency and computational performance compared to traditional von Neumann architectures.
- Quantum computing holds the potential to revolutionise AI by enabling faster and more efficient computation of complex AI algorithms. Quantum computers leverage quantum phenomena such as superposition and entanglement to perform computations exponentially faster than classical computers, offering significant energy savings and computational power for AI applications.