Data capital: Mining for gold in the data decade
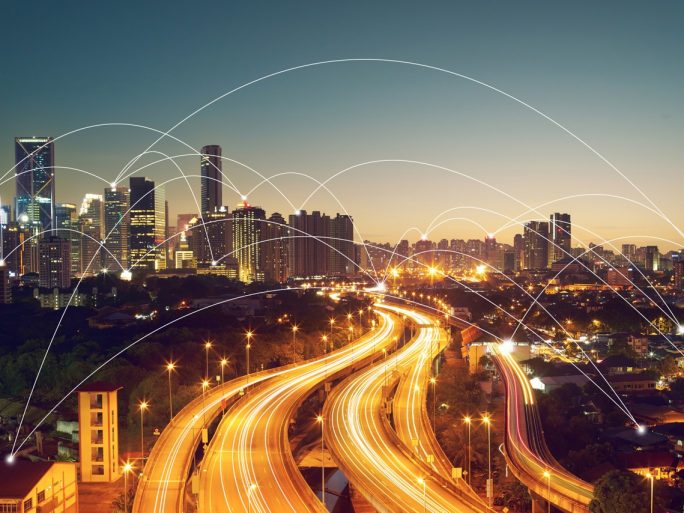
Across the globe, organisations large and small are starting to harness the power of data. Data capital will be viewed as one of a company’s most important assets, becoming an engine of commerce and innovation for the 21st century.
Whether it’s a car manufacturer, an inner-city artisan bakery or an international investment hedge fund, every business has several assets from which it can derive value.
These might be categorised as inventory (computers, kitchen tools, robotic manufacturing equipment), people, land, money, vehicles, investments and even good will. There are also assets that you can derive intellectual property from, and that you treat as a capital asset of the business.
Data capital is now being recognised as something akin to those other forms of assets, and more and more organisations are realising the opportunities it opens for them.
What is data capital?
Data assets may be centred around the customers that a business serves. People will have opted in, for example, to allow data that they generate to be used by the organisation. This will be familiar to anyone signing up to a mailing list or consenting to cookies on a website.
It might also generate non-person data, such as that produced from machines, stock or an understanding of processes in a given area of the market that they serve.
An organisation might also utilise a third party, such as a research or analyst agency, and gather external data on a market area. They would then typically blend that data with the data that they generate themselves, and the sum of the two would be how the company would consider data capital in the broader sense.
Is data now the most important asset a company has?
Data capital is becoming recognised as an increasingly tangible asset, and once organisations fully understand the power of the information they are gathering and how they then can use it to transform or improve, it’s going to become fundamental to the business world. So, if you are going to be in the business of generating and valuing data capital, how do you think about harnessing the power of it?
Agriculture and Industry 4.0
Fine in theory, you might say. But how does this map onto the real world? Let’s take the example of modern, disruptive agriculture to examine the process in more detail.
In this sector, AeroFarms is using data as a core tenant of its plan to upend and improve the traditional food manufacturing process. Its scientists use IoT (Internet of Things) sensors deployed throughout the growing environment to monitor over 130,000 data points every harvest and constantly optimise the growing system using predictive analytics to create optimum results.
The data generating and analysis this provides AeroFarms gives it the information required to do things more economically and more environmentally friendly, defying traditional growing seasons by enabling local farming at commercial scale all-year round, setting up facilities closer to population centres, and improving traceability. AeroFarms demonstrates that this is all done using 95% less water than field-farmed food and with yields 390 times higher per square foot annually.
Looking at the industrial sector, another good example of combining IoT and machine learning to produce actionable business insights can be found at Otto Motors.
The company makes robots that transport heavy objects in an industrial environment. These self-driving robots are equipped with IoT sensors, which inform how they navigate their way around the workplace. This provides the safest and most efficient working environment for both the robots and the human workers.
There might be ten, twenty or thirty of these robots simultaneously traversing the same space, so they use a set of machine learning algorithms to optimise the fleets’ performance.
Deep learning – the unknown unknowns
But what happens if you scale this up? Say for example a company like Otto Motors was asked to install a fleet of 100 robots for a customer, representing an exponential increase in the amount of gathered data and the complexity involved in managing a fleet of that size. Are the same algorithms still valid?
One solution would be to bring in the sub-branch of deep learning. This could overlay external data into the picture, such as data relating to ambient factory conditions like altitude, weather, temperature, pressure or humidity. Does the robot perform any differently when the factory floor temperature rises 5 degrees because it’s a hot summer’s day, as opposed to a cold winter’s day? If, with a larger fleet, the optimum routing around the warehouse means that the robots only make left turns, how does this affect wear and tear on individual components? Would this affect future design parameters or inform what software upgrades would maintain or increase robot performance?
These aren’t performance factors that are immediately obvious – what deep learning can in this instance reveal is what we call ‘unknown unknowns.’ Things that we didn’t even realise can affect the performance of a robot moving across a warehouse floor, but which are revealed when we overlay a complementary data set on top of the information landscape we have generated and gathered ourselves.
What technology do you need to tap into your own IoT enhanced data capital?
IoT data, which is the raw materials of this entire process, is highly unstructured in nature. The databases that you store it in are not traditional matrix databases – it exists in something called a data lake.
The storage and processing requirements of a data lake can be tiered based on desired response time. If data is being processed in real time, then you’re probably going to be using a combination of GPUs that can process data in parallel forms, closely associated with a lot of memory, which is where the data gets ingested and stored. The machine learning processes will require you to put those computing and storage devices close to where the data is being generated. This, in conjunction with the networks and infrastructure deployed to support a branch or remote location, can be defined as edge computing.
Technologies in the market that provide this capability are referred to as converged or hyperconverged. These platforms which combine virtualised compute, network and storage provide scalable platforms for cloud operating models, like those offered by the hyperscalers Google, Microsoft and Amazon Web Services.
What these hyperscalers tend to offer is a one-stop shop for everything you’d need. But the challenges with this approach are twofold. Firstly, a proof of concept (PoC) that starts small will have a set of well-defined costs associated with it. However, if the PoC is successful and leads to a full rollout, then the associated costs will scale rapidly. In this scenario there could well be a need to repatriate the application and dataset away from a hyperscaler environment. This is particularly true in edge computing environments, where cost and latency are incompatible with centralised storage and compute models, especially at scale.
Secondly, the hyperscaler environments are very much closed in terms of how you access the data; the way the APIs are managed are proprietary to the likes of Google and Amazon, and furthermore, are not interchangeable.
An alternative to this can be set up with the sort of technology that Dell Technologies provides – particularly in conjunction with our sister company VMWare. You can have a much more open and flexible approach to how you publish updates and access the APIs associated with the data and can use a multi-cloud operating model where you are always in control of the most appropriate environment to deploy the application and dataset. Today, many organisations recognise this as the most pragmatic, flexible and cost-effective approach to adopt.
Once a company has started making use of data in the ways described above it can start ascribing and appreciating the value of it.
Furthermore, once it’s been used to improve a business process the company could choose to sell the data or export it to other organisations. Through a broader ecosystem, or a kind of consortium, everyone learns from each other’s best practices, and as a result the data set is widened yet further.
The impact of marginal cost and the broader picture
What we now understand with IoT is that this technology moves the marginal cost of measuring things to near zero. For example, if you wear a smart watch the marginal cost of measuring your heart rate is near zero, because the function of doing so is built into the product.
IoT technologies have the potential to reduce the marginal cost of measuring anything to near zero. This leads to two outcomes: Firstly, the frequency of measurement will increase because there is no penalty for doing that. Secondly, you’ll have a much higher volume of data from which to extract value.
So, if we measure something more frequently, we gain a greater accuracy around how that entity is behaving. The data is then put through a machine and/or deep learning process so that we can generate actionable insight from it. It is this potentially seismic impact in every industry and every sector that explains why data can be considered as a capital asset and why companies large or small should be assessing the data capital they own and how they best deploy this asset to either gain competitive advantage, reduce cost, open up a new market opportunity or….all of the above. Data capital truly is a gold mine in the information age.